Python is one of the fastest growing programming languages, with reported usage rising 20% in the last five years. More than half of developers use Python, with another 8% planning to adopt it in the next year. It holds its own against languages like JavaScript, Java, Kotlin and Go. In fact, I can almost guarantee that your company is using Python for something right now.
Yet for all its popularity, Python is often misperceived as slow, clumsy and limited to small projects. The reality is, Python is a powerful and flexible language. It enables development teams to rapidly develop robust, elegant solutions to tough problems. In this article, I’ll bust four myths about Python and explain why it’s so valuable.
Myth 1: “Python is just a scripting language.”
A scripting language operates atop an existing software system to extend its functionality. However, the term is more often used as a sneaky pejorative to imply limits on a language’s usefulness. The speaker often wants to frighten others into using the “real” language they prefer instead.
While Python excels in the role of a literal scripting language, it is by no means limited to this functionality. We can compare it to the Java programming language, long a staple of serious software engineering. Both languages sport the ability to interface with the network and operating system; for this reason, Python is a mainstay in server and network automation. Extensive standard libraries and development ecosystems enable both to excel in almost any practice area. Python is very much Java’s functional equal in all regards.
Myth 2: “Python is slow.”
Because Python is an interpreted language, there is some truth to this. There are many implementations of the Python language and most are slower than languages like Go and Rust. For this reason, some developers are hesitant to use it for performance-critical projects, such as API backends and big data pipelines, yet Python actually excels at both!
Not all Python interpreters have the same performance. One of these, PyPy, implements a just-in-time compilation strategy similar to the Java JVM and thus achieves Java-like performance.
Python also supports binary extensions, which allow communication with compiled C code, typically to handle CPU-intensive operations. Libraries like numpy, Modin and Pillow use them to boost performance. Python can even interface with code from other languages via the CFFI binary extension. A few tools can even compile parts of your Python code to machine code in advance.
Finally, the default Python interpreter, CPython, is undergoing significant improvement. Python 3.11 is showing performance improvements between 10-60% over 3.10 and even higher compared to Python 3.9 and earlier. The notion of CPython being sluggish is starting to become a thing of the past.
Myth 3: “Python is single-threaded.”
A single Python process cannot use more than one CPU core, because of the language’s Global Interpreter Lock (GIL), which actually improves interpreter performance. Nonetheless, Python is fully capable of parallelism and concurrency, which are crucial tools for creating responsive user experiences and performing complex computations.
Asynchrony and traditional threading make concurrency possible in Python. Parallelization is achieved through multiprocessing and extended via libraries like Ray, Dask and JobLib, all of which bypass the GIL. Binary extensions can also be used to parallelize expensive operations.
If anything, the delineation between parallelism and concurrency gives developers more control over when and how to use each.
Myth 4: “Python is weakly typed”
Static typing, specifying the data types for variables, is familiar for many developers. For them, Python’s dynamic typing system can feel a bit like working without a safety net at first. Once you’re used to it, however, it is one of Python’s greatest strengths.
Values in Python are still strictly typed. Unlike weakly typed JavaScript, Python won’t let you do surprising things like adding strings and numbers. However, dynamic typing means that code interacts with an object on the basis of capability, greatly simplifying architecture. Once a developer learns to use Python’s dynamic typing to their advantage, it can save them days of effort.
Should I Use Python?
Selecting a language for a project is not a simple process. It helps to consider both the technical needs of the project and the existing team’s skills and knowledge. Python isn’t necessarily the answer to all your problems, any more than Java is, but it certainly bears consideration. Anything you can do in Java or another programming language, you can do in Python, and usually with significantly simpler code. Testing, style and code quality are easily implemented, thanks to tools and standards oriented around evolving best practices. All this saves significant development time, both up front and in maintenance.
About the Author
Jason C. McDonald is a Principal Consultant at Sparq. As a Python expert, he’s a speaker and the author of “Dead Simple Python” (No Starch Press). When he’s not coding, he’s usually writing fiction books, playing piano, or spending time with his mother and two dogs.
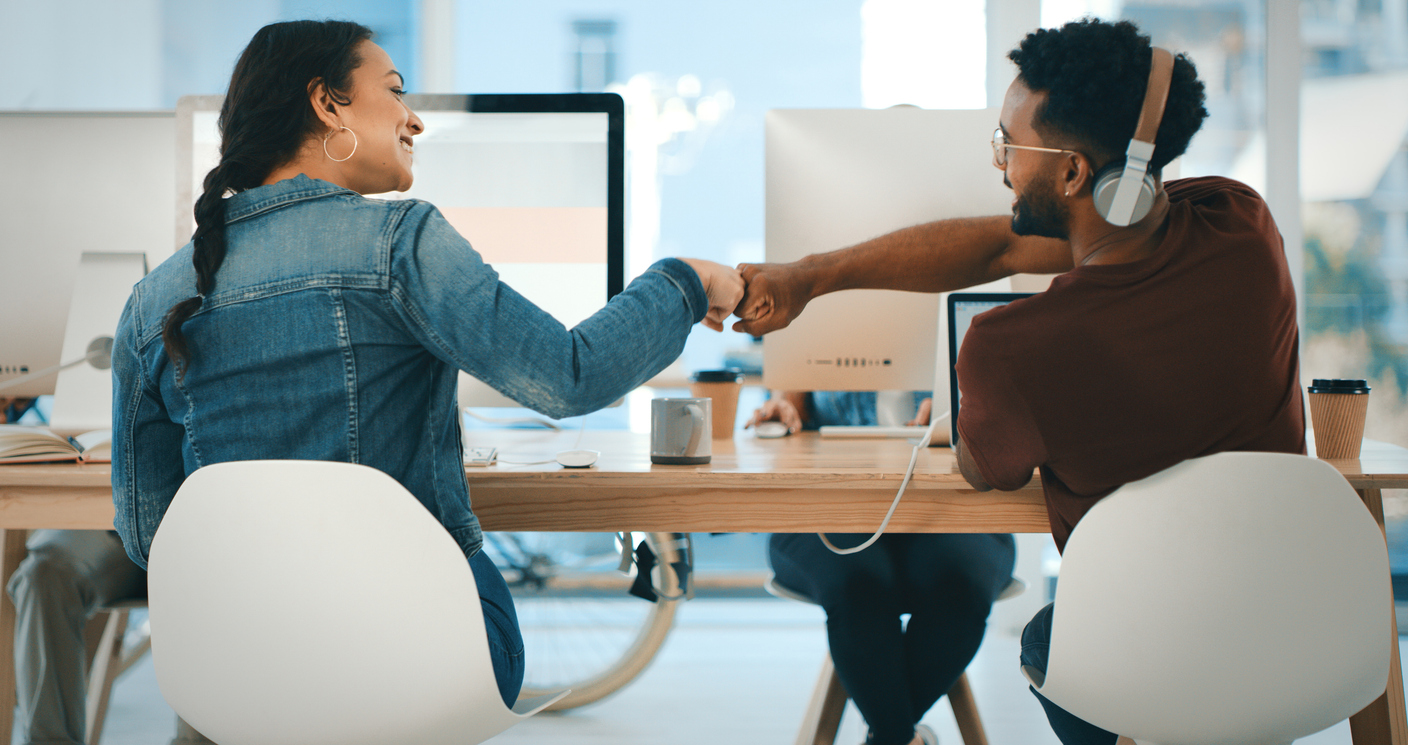
Soft Skills for Tech Leads: How They’ll Help You Excel at Your Role
If you manage a team, you already know that this experience can be as challenging as it is rewarding. At Sparq we have different management roles and one of the most essential is the Tech Lead position. In addition to being seasoned developers, Teach Leads need to be able to mentor up and coming talent. One of the ways they do that is by utilizing soft skills. In this article, Delivery Lead Rodrigo Trelles explores the most important soft skills to be a successful Tech Lead.
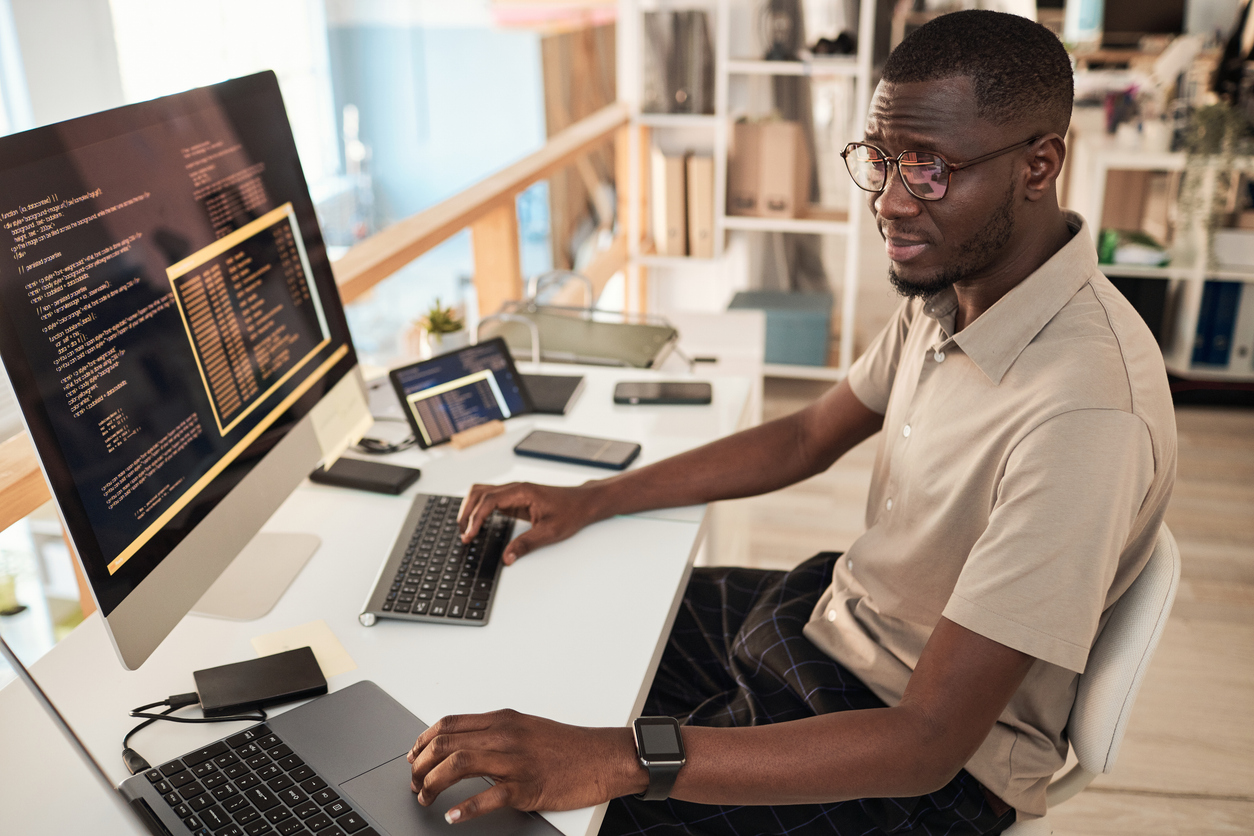
Exploring the World of a Site Reliability Engineer (SRE)
Technology is advancing rapidly and the new advances introduce new roles and responsibilities. Among the most significant roles is the Site Reliability Engineer (SRE). This article will explore the role of an SRE, how it differs from a software developer and the essential skills required to succeed in the field.
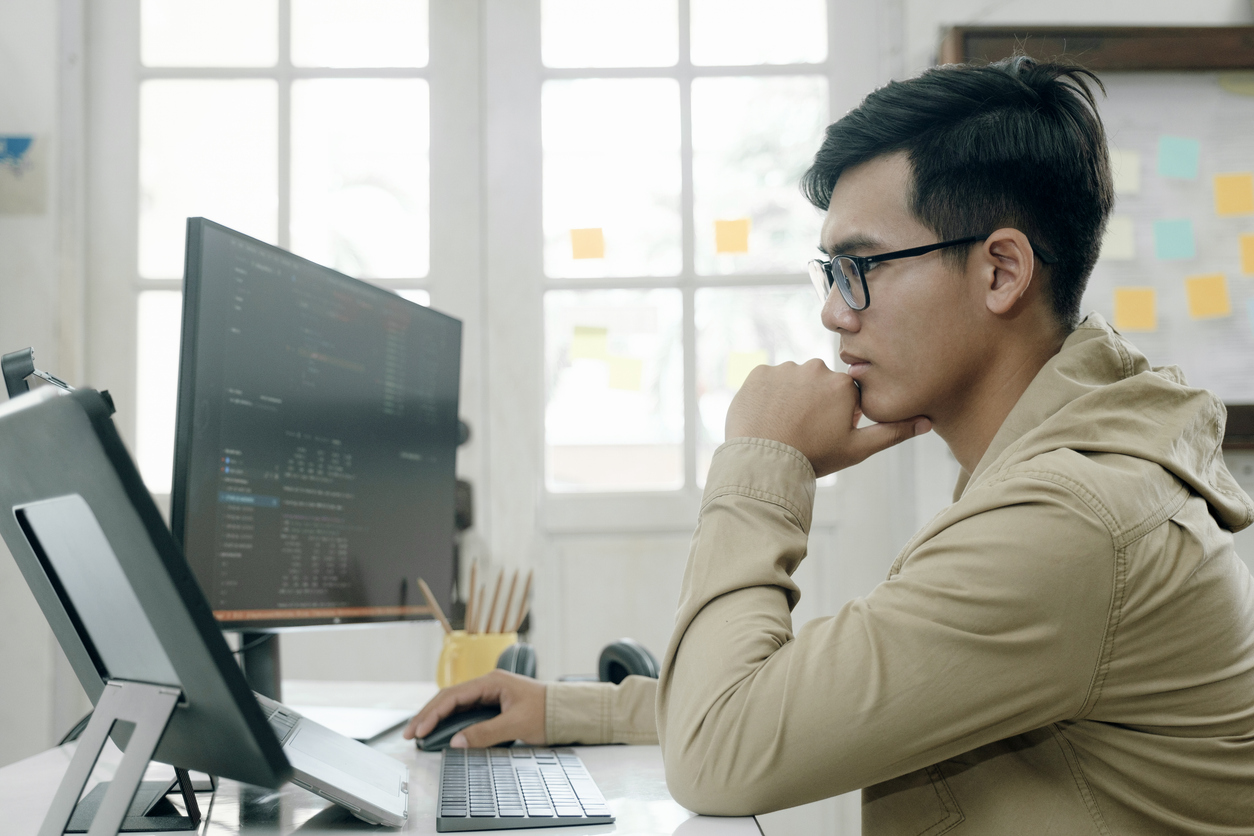
Automated Testing Applied: How This Practice Can Help Developers Across Projects
In this article, Technical Lead Pablo Carballo shares how automated testing practices have made his team's work more efficient and diverse.
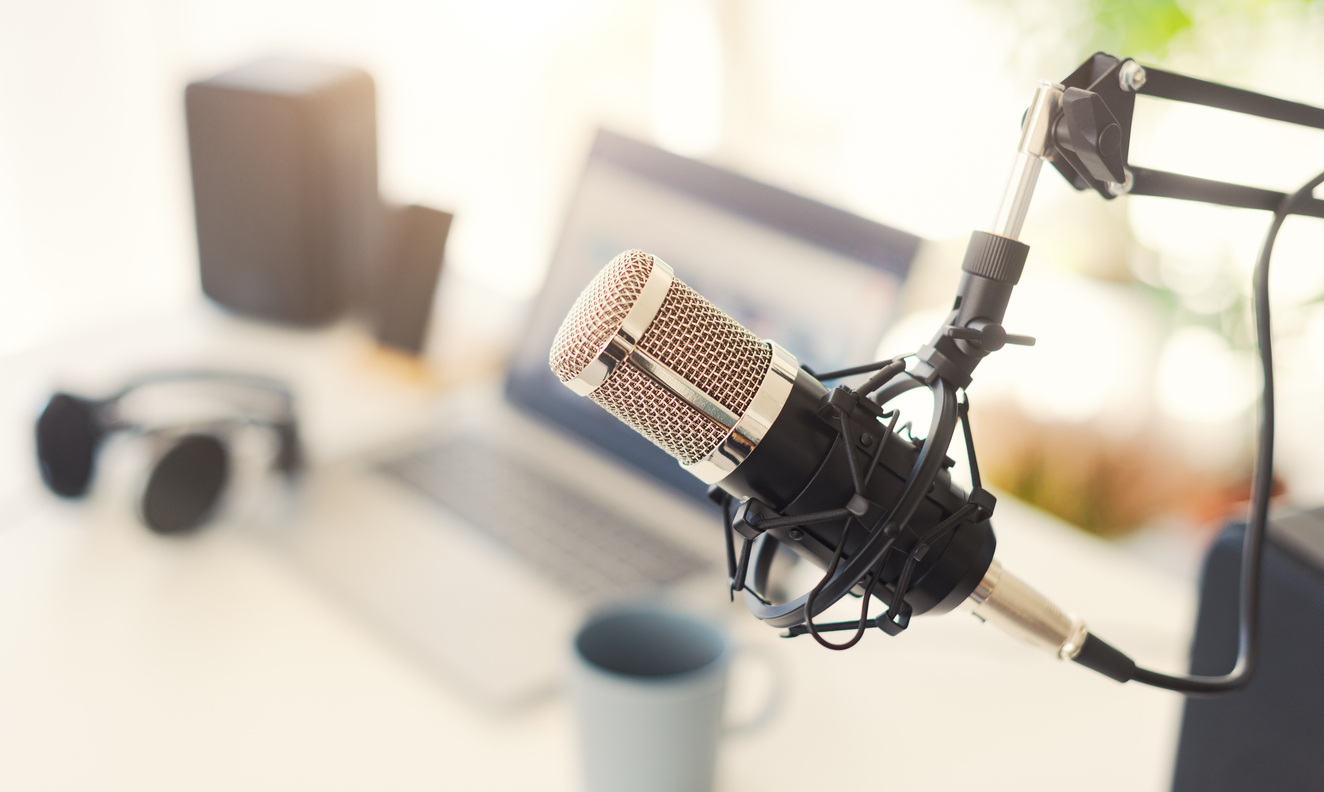
Inside the Minds of Digital Innovators: Highlights from OctoTalks Podcast
Creating exceptional digital experiences involves a blend of creativity, strategy and technology. Octobot, a company that was recently acquired by Sparq, hosted a podcast that delves into these elements by showcasing the visionaries who dream up and build these experiences. In this blog post, we want to share some highlights about the conversations and invite you to give it a listen!