In an era where artificial intelligence (AI) promises to revolutionize industries and redefine competitive landscapes, generative AI stands out for its ability to create new content, from text to images, videos and beyond. This technology holds immense potential for businesses across industries, promising to revolutionize product development, marketing, customer service and more. However, the effectiveness of generative AI is inherently tied to the quality of data it is trained on. Despite the enthusiasm surrounding these advancements, many companies find themselves unprepared to harness the full capabilities of generative AI, primarily due to inadequate data infrastructures. This article explores the pivotal role of high-quality data in generative AI efficacy, examines the preparedness of companies for adopting these technologies and outlines essential steps for building a robust data foundation.
The Foundation of Generative AI: High-Quality Data
Generative AI operates by learning from vast datasets, identifying patterns and generating new outputs based on the learned information. The diversity, quality and relevance of the training data directly influence the AI’s ability to produce accurate, innovative and unbiased content. High-quality data is characterized by its completeness, accuracy, diversity and relevance. When generative AI systems are fed with poor-quality data, the consequences can range from generating inaccurate outputs to perpetuating or amplifying biases, thus diminishing the technology’s utility and potentially harming the company’s reputation.
Moreover, the specific challenges of data quality for generative AI include ensuring a diverse and inclusive dataset that represents a wide range of perspectives and avoiding data that could lead the AI to generate harmful or biased content. Ensuring the data is up-to-date is also crucial, especially in rapidly changing fields where outdated information could lead to irrelevant or incorrect outputs.
Assessing Company Preparedness for Generative AI Adoption
The rush to adopt generative AI technologies often exposes a critical gap in many companies’ data strategies. Several factors contribute to this gap:
- Data Silos: Fragmented data ecosystems within organizations make it challenging to aggregate the comprehensive datasets needed for effective generative AI training.
- Data Governance and Quality: A lack of rigorous data governance frameworks leads to inconsistencies, inaccuracies and gaps in data, directly impacting the quality of AI-generated outputs.
- Ethical Data Sourcing and Bias Mitigation: Ethical considerations in data sourcing and the need to mitigate biases in AI-generated content are increasingly recognized as critical elements of AI strategy. Companies must ensure their data collection methods are ethical and that datasets are diverse and representative to prevent biases in generative AI applications.
- Regulatory Compliance and Data Privacy: As regulatory frameworks for AI and data privacy continue to evolve, companies must navigate an increasingly complex legal landscape. Ensuring compliance with regulations such as GDPR, CCPA and others while leveraging data for generative AI poses a significant challenge.
- Scalability of Data Systems: Many companies lack data systems that can scale effectively to meet the demands of generative AI applications. As generative AI models become more sophisticated, they require increasingly large and complex datasets for training, necessitating scalable data storage, processing and analysis capabilities.
- Data Annotation and Labeling: Generative AI models, especially those used in supervised learning, rely heavily on well-annotated and labeled datasets. The lack of accurately annotated data can significantly hinder the model’s training process and affect the quality of the generated outputs. Companies often underestimate the time, resources and expertise required for effective data annotation.
- Real-time Data Processing: Generative AI applications in areas such as customer service or personalized content creation require the ability to process and analyze data in real-time. Many businesses struggle with integrating real-time data processing capabilities into their existing data infrastructure, limiting their ability to deploy dynamic generative AI solutions.
Building a Strong Data Foundation for Generative AI
To leverage generative AI’s full potential, businesses must undertake a comprehensive approach to strengthen their data foundation. The following steps are crucial:
- Comprehensive Data Audit: Begin with a thorough audit to understand the current state of data assets, identifying gaps, silos and quality issues.
- Enhance Data Governance: Implement robust data governance policies that address data quality, privacy, security and ethical considerations specific to generative AI. This includes establishing clear guidelines for data collection, storage, usage and the continuous monitoring of data quality.
- Cultivate a Data-Driven Culture: Promote a culture that values data literacy and ethical AI use across all levels of the organization. Training and development programs can empower employees to leverage generative AI tools effectively and responsibly.
- Invest in Data Integration and Management Tools: To break down silos and create a unified data ecosystem, invest in advanced data integration, management and storage solutions that can handle the scale and complexity of datasets required for generative AI.
- Prioritize Ethical and Diverse Data Collection: Ensure that datasets are not only large and comprehensive but also diverse and ethically sourced. This helps in training generative AI models that can generate unbiased and representative outputs.
- Develop Technical Infrastructure: Upgrade the technical infrastructure to support the intensive computational requirements of training and running generative AI models, including high-performance computing resources and cloud storage solutions.
- Partner with Experts: Collaborate with data analytics experts, AI ethicists and legal advisors to navigate the complexities of generative AI implementation, from ensuring data quality to addressing ethical and legal considerations.
- Adopt an Agile Approach to Data Management: As generative AI technologies evolve, so too should your data management practices. An agile, flexible approach allows for the rapid incorporation of new data sources, tools and methodologies to keep pace with advancements in AI.
The successful adoption of generative AI hinges on a company’s ability to build a robust data foundation that emphasizes quality, diversity and ethical sourcing. As businesses strive to leverage generative AI’s transformative potential, addressing the critical gap in data strategies requires a multifaceted approach that includes scalable data infrastructure, rigorous data governance and a culture of data literacy and ethical AI use. By prioritizing these elements and fostering interdisciplinary collaboration, organizations can not only overcome the challenges associated with generative AI but also unlock innovative opportunities to position themselves at the forefront of this technological revolution and creating lasting value.
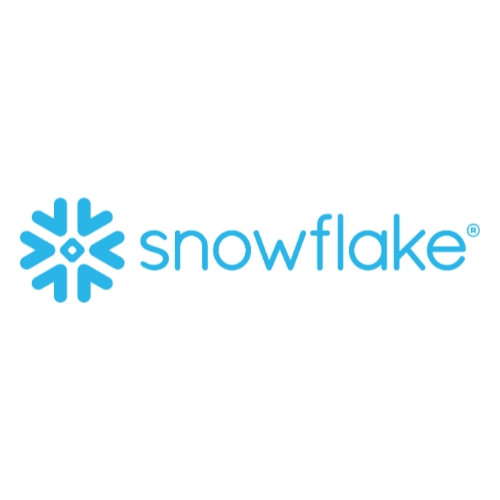
Snowflake Summit 2025 Announcements
Snowflake Summit 2025’s latest announcements made it clear: the path to genuine AI-driven impact hinges on frictionless access to data, the ability to act on it with clarity, and absolute confidence in its protection. Learn more about how they're making that happen for customers in this article.
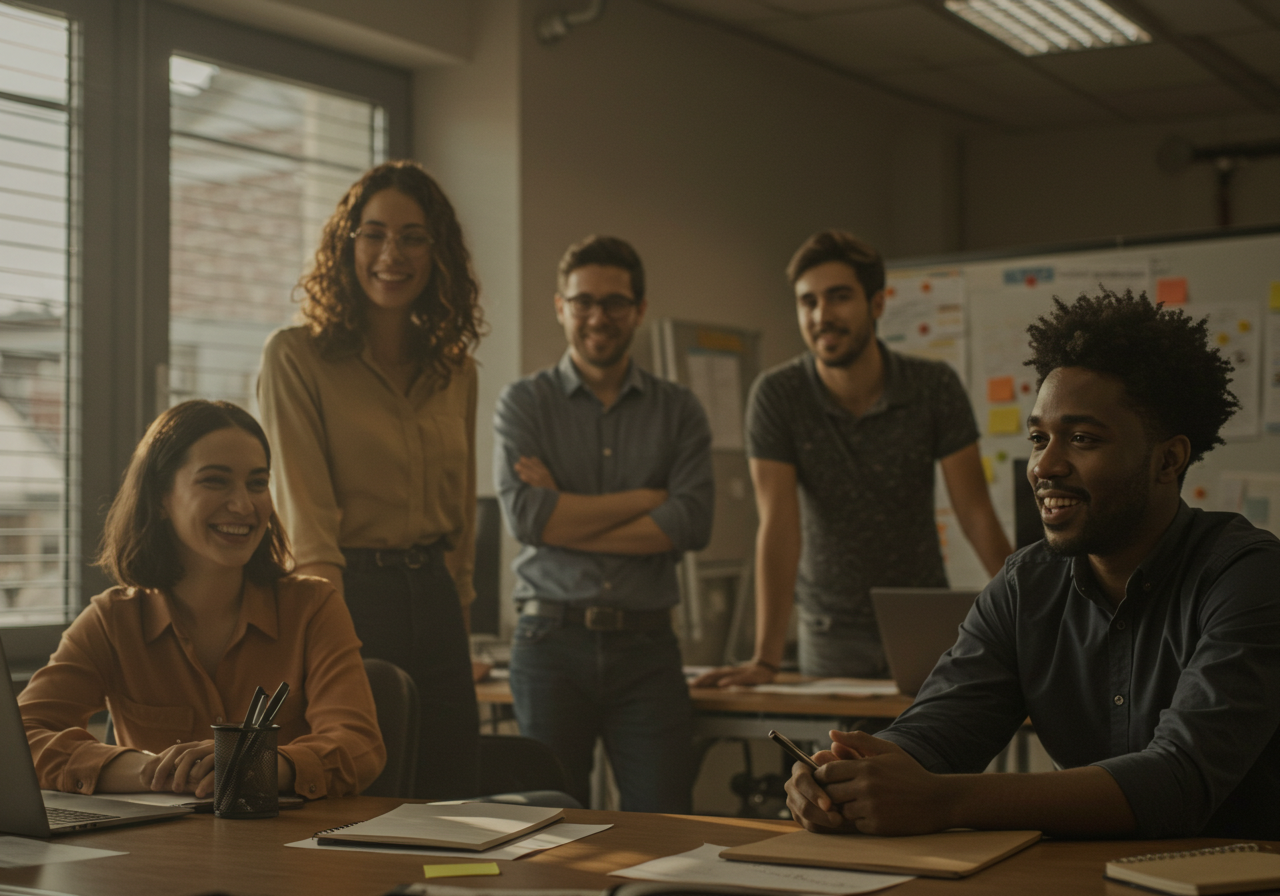
How ChatPRD Helps Build Better Stories (and a Stronger Team)
When user stories are vague, it slows down delivery, trust, and momentum. This article by Senior Product Strategy Consultant Traci Metzger shows how she used a lightweight, AI-guided system (ChatPRD) to write clearer, developer-ready requirements that actually accelerated execution.
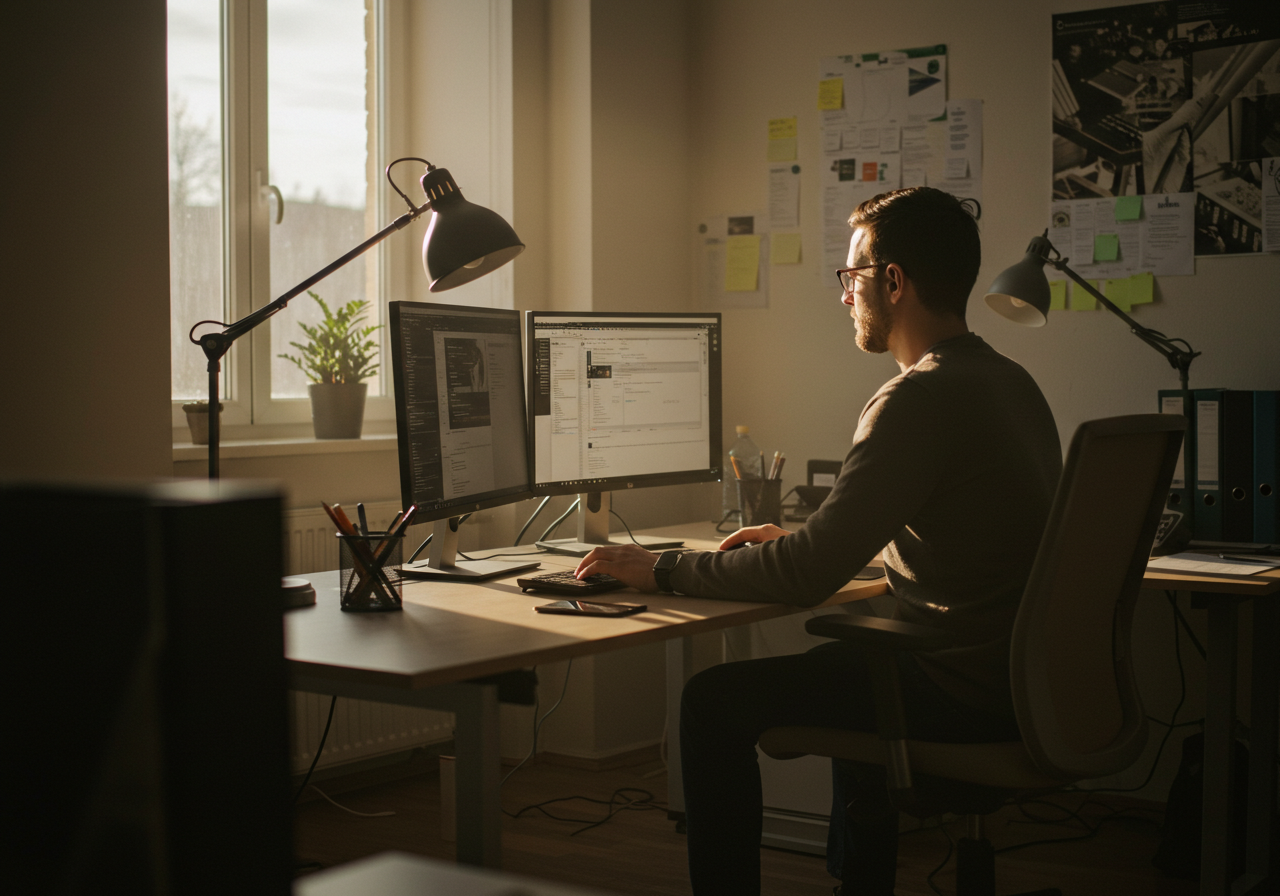
QA in the Age of AI: The Rise of AI-Powered Quality Intelligence
As organizations push code to production faster, respond rapidly to new customer needs and build adaptive systems, the expectations on quality have changed. It's no longer enough to simply catch bugs at the end of the cycle. We’re entering an era where quality engineering must evolve into quality intelligence and organizations adopting quality intelligence practices are reporting measurable gains across key delivery metrics. Learn more in this article by Principal Engineer Jarius Hayes.
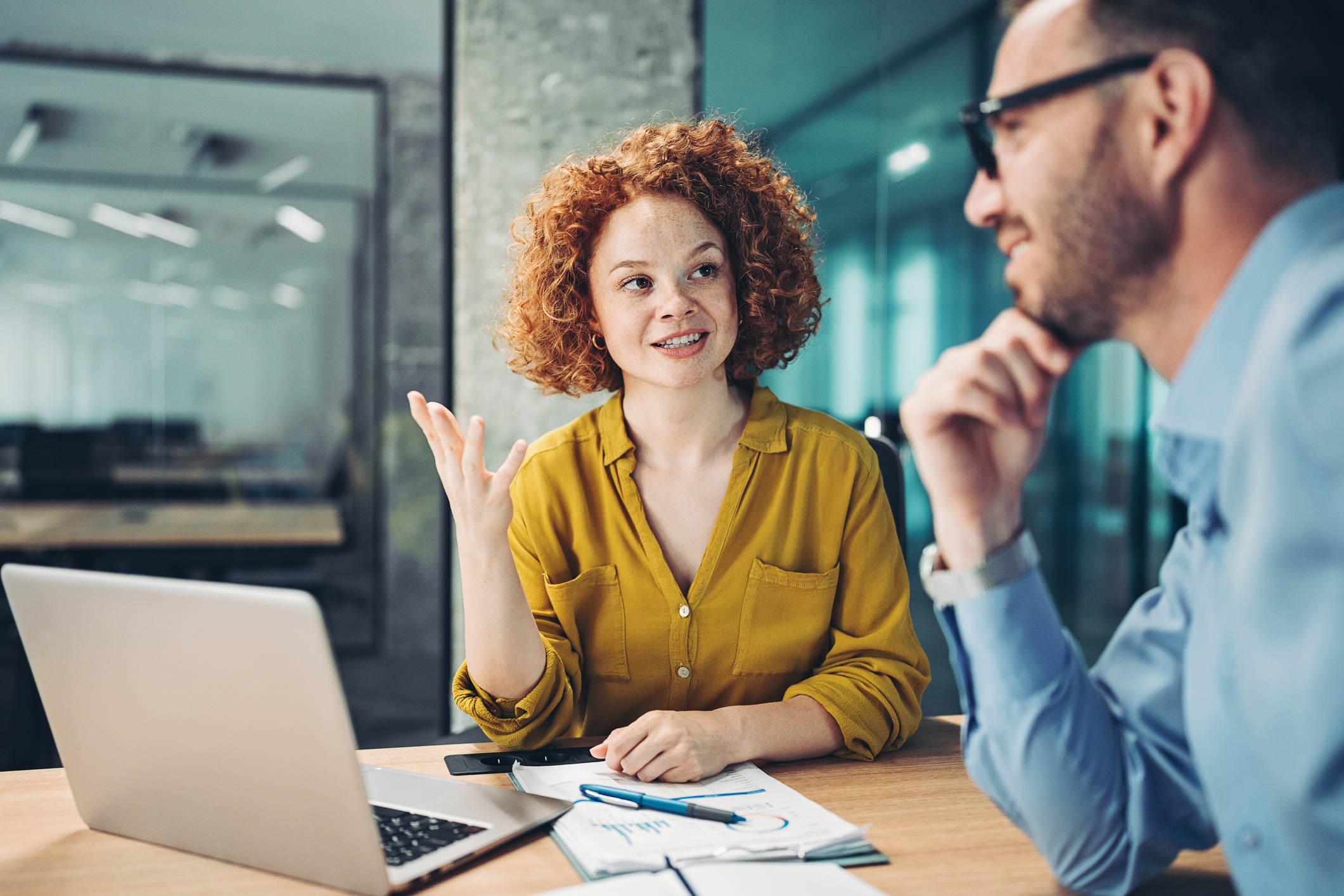
Operational Efficiency in the AI Era: What Matters and What Works
Ever wonder how leading teams are cutting costs without cutting corners? Hint: it starts with AI. In this article by Principal Delivery Manager Kabir Chugh, learn how AI is powering smarter ops, faster deployments, and measurable savings across industries.